How Predictive Analytics Can Show When Your Customers Will Leave
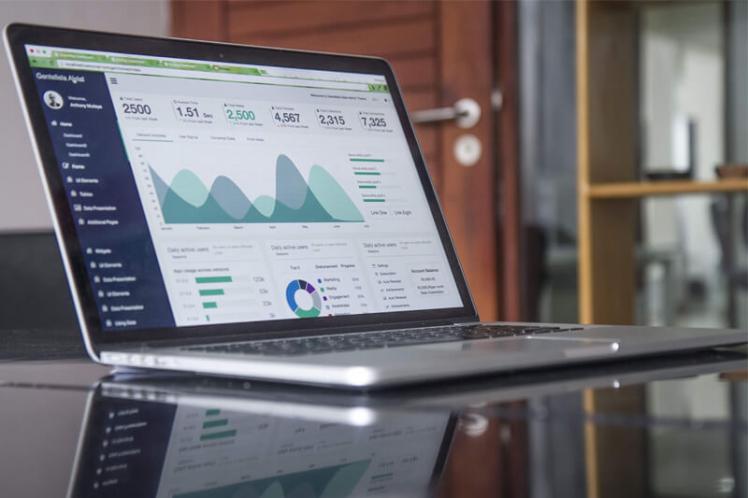
Question: how many of your customers are going to leave you?
Answer: all of them!
No, it’s not a trick question – all of your customers will leave you, sooner or later. However, this shows the importance of being able to predict the likely timing of their departures.
Fortunately, there’s a branch of statistics which can do just this. Survival analysis focuses on the analysis of time – which could be the survival times of patients, intervals between equipment failures, or lifetimes of customers.
Survival analysis has been designed to overcome an obvious difficulty –by definition, you don’t yet know when your current customers are going to churn. They might leave tomorrow, or they could stay for many more years. You only really know the lifetimes of your past customers, yet you still need to consider both churners and non-churners. Why is that? Because, on average, non-churners will tend to have longer lifetimes; so if you exclude them, you will be understating the longevity of your customer base.
The situation of not knowing when current customers are going to churn is known as censored data. Survival analysis techniques have been designed to handle censored data, in order to describe and predict the lifetimes of people, things and customers.
The first step in survival analysis is to describe the rate of decay being experienced by your customer base, as a function of time since recruitment. This can identify points in the customer lifecycle where churn rates tend to increase. For example, mobile phone customers recruited on fixed-term contracts typically churn ‘in droves’ as soon as their contractual period ends. The descriptive survival analysis shows this occurrence as survival rates ‘falling off a cliff’. The technique can also identify less obvious risky moments, such as high attrition rates when phones approach a certain age.
Next, you can measure how the survival patterns vary between subsets of customers, such as those recruited via different channels or belonging to different segments. This will highlight the factors that have the strongest influence on customer lifetimes and may help you to make product improvements or introduce marketing interventions to retain groups that are at risk of churn.
The ultimate application is to predict the decay curves for individual customers. While this can never pinpoint that the customer is going to churn on a specific future date, it does identify when they become more likely to leave than to stay. By knowing that a high-value customer is likely to reach this critical stage in six months’ time, you are able to select an appropriate marketing treatment designed to retain them and time your intervention to have maximum effect.
By seeing that another customer is already likely to churn and that their departure is unlikely to be prevented cost-effectively, you can avoid trying to keep them. Instead, you can apply your retention budget to customers who could potentially be saved and are also sufficiently profitable to justify taking action.
Survival analysis is an excellent example of statistics being harnessed by predictive analytics to create new insights and improve your marketing.