Learn to Work With Artificial Intelligence (AI)
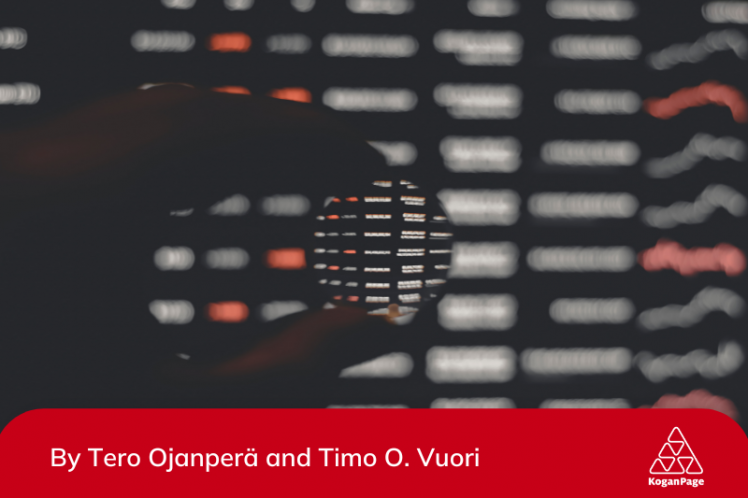
The following is an edited extract from Platform Strategy.
Change won’t happen overnight. Your employees and yourself have different expectations and assumptions about new technology. Although everybody might agree that the change is inevitable in the long term, they might even resist AI and platform business models in the short term. Your challenge is to turn fear into energy.
For an organization to work, it needs to have clear targets, roles and decision-making rules. The same applies to AI. If AI is in an assisting position, but employees think it should decide, bad things might happen. Or, if members of the organization expect AI to decide, but managers don’t trust it and make their own decisions, things might go south.
So, define what you want from AI. Are you seeking advice on different alternatives or automated decisions? Or do you want AI to learn new parameters that influence decisions? You should understand the context where AI is being used and remember that AI learns from new data. And thus, AI can change the way an organization works as it learns more. Each of these steps leads to a different organizational design from a human point of view. The goal is not to automate everything but to design a set-up where AI’s potential is matched with the task at hand.
Use these four steps to learn to work with AI
Four steps for how to learn to work with AI:
- Allow AI to make recommendations, but let humans decide
- Allow AI to make automated decisions based on pre-determined criteria
- Use AI to create new criteria for a human to decide
- Allow AI to make autonomous decisions based on its learning
1. Allow AI to make recommendations, but let humans decide
In this scenario, AI is your best adviser, but it won’t decide on your behalf. This scenario is, therefore, a safe first step, as you are learning to work with AI.
AI can analyze tons of data you couldn’t go through in your lifetime in a couple of seconds. Based on pre-determined criteria, AI recommends various options that humans can then choose. As humans get to choose, they maintain a sense of control and are likely to enjoy working with AI.
Imagine that AI chooses a team to perform a specific task. In this scenario, based on an approach developed by IBM, a human defines parameters that are relevant for the performance of the team. This human reflects what skills the team should have to succeed in its task. They rely on experience and intuition to identify the needed characteristics. The parameters could be, for example, that at least one team member should have each of the following attributes:
- Five years of experience in cloud programming.
- Five years of experience in the industry of the client.
- A pre-existing relationship with the client.
- A business degree.
- Fluency in French.
- Located in central Europe.
The human would define these attributes for the AI system. The AI would search for suitable employees throughout the firm’s databases and suggest an optimal team. AI could use various approaches for recognizing the needed skills, in addition to formal CV and skill databases, such as the textual analyses we discussed above. The AI might suggest, for example, a three-person team:
- Person 1
- Experienced cloud programmer from the US
- Person 2
- A marketing expert who has worked with the client for over five years
- Fluency in French
- Located in central Europe
- Person 3
- Recently hired MBA from the Singapore office
Seeing this suggestion, the human could decide whether to accept or reject the AI’s proposal. For example, the human might reason that it would be ineffective to have people from three different time zones. Hence, he or she might reject this proposal from the AI (and potentially add a location constraint in the criteria).
If the human rejected the AI’s proposal, the AI could then search for an alternative combination. The AI could also be programmed always to suggest, say, three alternatives from which the human could then choose. AI assists in the decision-making but doesn’t decide.
In what decisions could you use additional input from AI? Consider the variety of choices made every day in your organization. Which of those choices contain criteria and attributes that could be programmed for AI? Should you contact a vendor to create an AI system for you to augment these decisions?
2. Allow AI to make automated decisions based on pre-determined criteria
In this scenario, a human decides the criteria for a decision. Based on those, AI can make an automatic decision. To continue our team selection example, a human would define the criteria used for selecting the team, and AI would automatically determine the team composition and schedule the team for work.
By adopting this approach, you gain efficiency. You can use this approach once people have learned to trust the AI’s recommendations in the first step. After you notice that you or your team has accepted the last 20 recommendations from AI, you could conclude that it’s time to let the AI work autonomously.
A challenge in this approach is that the set of criteria created by the human might be incomplete. For example, maybe there is a personal conflict between two employees. Therefore, they should not be included in the same team. However, if not listed as a criterion, the AI would not consider this conflict, even if everyone in the company knew about the conflict. It would help if you, therefore, thought also how you could maximize the AI’s learning.
3. Use AI to create new criteria for a human to decide
AI could observe teams’ outcomes at specific tasks, analyze why they fail or succeed and discover new criteria for selecting the teams. These criteria could be understandable by humans but could also be very complex and hard to explain.
For example, when recommending an optimal team, AI would learn the team members’ optimal attributes instead of relying on the human definition. AI could do this by analyzing the performance of (relevant) past projects and comparing them to project member attributes, the project’s goal and various other characteristics related to the project. Essential here is that instead of relying on human intuition and pre-established ideas of what makes an excellent team, the AI would learn which attributes predict team performance. Through such an approach, Google discovered how psychological safety is one of the key factors contributing to team performance.
Some other characteristics recognized by AI could involve those that are intuitive for humans, such as client industry and country. Also, they could include others that are less intuitive, such as the season (summer, autumn, winter, spring) or the height of project team members.
If AI has access to project conversation data, it could infer team characteristics from it. Without defining the criteria, it could conclude, for example, that teams using language that leads to psychological safety perform better than others.
Once AI had identified the relevant attributes, it would search for employees that fit the characteristics. After recognizing them, it would suggest one or a few alternatives for the humans to decide. The human would then make a choice.
A challenge in using ‘AI to create the criteria but letting humans decide’ is that the human may not understand why the AI is suggesting the teams it is suggesting. The options might seem counter-intuitive or wrong for the human.
It is difficult for humans to act on recommendations that do not make sense to them. However, sometimes it is beneficial to do so. For example, a top-notch hedge fund, Renaissance, uses AI extensively and sometimes its employees do not understand the AI’s recommendations. Still, they profit by trading securities based on non-intuitive anomalies that were detected by machine learning but are hard to explain.
Hence, this approach requires courage and trust in the AI from the decision-maker.
To reduce the need to trust AI blindly, you can invest in explainable AI. This refers to modules that make AI’s recommendations more understandable for people. For one, where feasible, you should select algorithms that contain explicit logic for their choices. In addition, it’s worth creating an explanation interface that provides the rationale for the user.
Several new techniques for making AI more explainable are being developed and you should keep track of the most advanced solutions. As an executive, you need to recognize that it might be worth the additional investment.
4. Allow AI to make autonomous decisions based on its learning
As the last step, you let AI learn and make autonomous decisions. For example, AI could observe the teams’ outcomes at specific tasks and detect why they fail or succeed. It would adjust its criteria accordingly for selecting the teams based on this. It would then automatically assign team members for the tasks that followed.
This approach has at least two substantial benefits: efficiency and freedom from human bias. You gain efficiency as there is no need to have a human make sense of the alternatives or fight political battles. You are free from human discrimination, as there is no human who would override the AI’s recommendation. In addition, as the AI is learning from the performance data, it is likely to develop a superior understanding of factors influencing team success. It can therefore create stronger teams than humans could.
The downsides of this approach relate to the loss of human visibility and control. The AI likely learns beneficial patterns and makes optimal decisions. However, when there is a situation that qualitatively differs from the past, AI might make wrong recommendations while people would see the obvious implications.
In addition, as more thinking responsibility and control are given to the AI, there are fewer opportunities for humans in your organization to grow into managerial roles. Without AI, lower-level managers learn the business logic by making repeated decisions. But when those decisions are fully automated, those managers lose their chance to practise.
However, the benefits often outweigh the risks and it is useful to start building this approach. You could start by automating simple decisions. What decisions could you already automate to a learning AI? Once you gain familiarity and begin trusting the AI, what would be the next set of automated decisions handed to a learning AI?
Key takeaways for your organization
To conclude, we have outlined different ways of deploying AI in your organization. There is no right or wrong, as it depends on the context.
Many people think the ultimate goal is to automate everything. That might not be desirable or sometimes not even allowed from a legal point of view. AI might not know the context of the decision, and you thus still need human input. The good news is that as a human, you can decide the level of input you will have when planning how to organize your company for AI.