Using Business Analytics in Supply Chain Systems
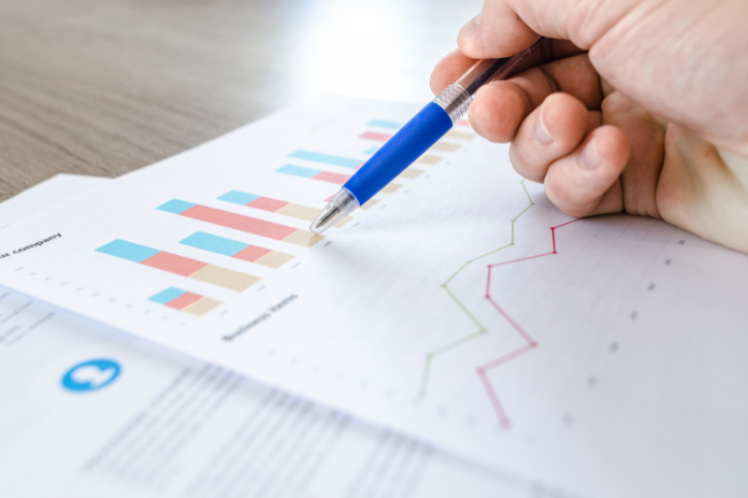
Different business analytics approaches are used by organizations to create values from data available to them.
However, organizations are not operating in isolation as they form links and connections with many other organizations who all seek to create value from data and maintain their competitive position in the current market.
Over the years, various aspects have been modelled and analyzed in the supply chain and a number of business analytical techniques have been adopted and reported having benefited the supply chain.
Following current technological developments, many organizations are embracing the use of analytics. It is therefore relevant to understand what is captured under the definition of Business Analytics (BA) in the context of supply chain.
Tipi (2021) has noted that BA is seen as a collection of tools, approaches, procedures, that are all coming together to engage in collecting relevant data and information, evaluating this using the most appropriate techniques, and providing suggestions for a potential solution based on the problem on hand.
Considering the use of BA in the context of supply chain, where various organizations are coming together to form the chain, it can be assumed that the challenge comes from integrating analytics across individual organizations forming part of the chain. Therefore, a relevant question to note is how BA approaches are being developed and implemented across the supply chain that brings value not only to an individual organization, but to the entire supply chain.
A number of analytical approaches (see Figure 1) have been categorized under descriptive, predictive and prescriptive analytics (Wang et al., 2018), where other authors are adding to these categories: inquisitive analytics and pre-emptive analytics (Sivarajah et al., 2017); or as mentioned in Sukhobokov (2018) executive analytics and reflexive analytics on top of descriptive, predictive and prescriptive analytics.
Further details of each individual category are depicted in the following section, a review of Business Analytics approaches and represented in Figure 1, with an emphasis on prescriptive analytics.
It is relevant to note that the key challenges are in assessing the need for analytics, selecting the most appropriate tools and techniques to implement these in the supply chain as well as create the opportunity to maintain and update the models used over a period of time. These challenges are further depicted in the discussion section.
Figure 1: Business Analytics for supply chain (Source: further developed from Tipi, 2021)
A review of Business Analytics approaches
Business Analytics applications are not only in the supply chain, as they can be noted in a number of other areas such as accounting, financing, budgeting, engineering, healthcare, human resources, manufacturing, marketing, service operations, sports management, legal studies, libraries, military, transportation, port operations, retailing, education, travel and tourism and others (Tipi, 2021).
A range of software packages have been adopted and used when implementing analytics from classical spreadsheets with the use of Microsoft Excel and Access, to Minitab, SPSS, with software for optimization such as AIIMS AMPL, Frontline, GMAS Lindo (Sharda, et al. 2018) and others such as Apache (Flink, Hive, PIG, Spark and Storm), AWS, Azure, C/C++, Caffe, Cognos, Dataiku, Hadoop, Java, Matlab, Python, R, Scala, Stata, Tableau, Tensoflow, Teradata, Tibco to name just a few from Stanton and Stanton (2020) study.
For any of these, there is the expectation to have the skills to use, maintain and benefit from the value they may offer.
Descriptive analytics
Enquiries that are using descriptive analytics are those that are looking to determine what happened, or what is currently happening in a particular situation. The most common techniques dealing with descriptive analytics are reports or ad-hoc reports, queries (Wang et al., 2018), dashboards, OLAP-analysis (Sukhobokov, 2018), performance measures evaluations, mapping, visualisation (Souza, 2014; Tipi, 2021).
Data for this type of analyses may come from the organization’s own database, or from barcodes, RFID, ERP system, or other visualisation tools that are internal or external to the organisation.
These type of analyses are frequently used with organisations and across the supply chain and, in general, the skills required to engage with these type of analyses do not necessarily present major challenges.
Predictive analytics
This approach tends to ask questions on what may happen in the future, how it may happen, or if a particular trend may continue in the future.
The source of data for this type of analysis may be similar with the data from descriptive analytics. Still, data in this case can also be predicted based on employing analytics and by using historical data.
Lepenioti et al. (2020) consider under the category of predictive analytics models such as probabilistic models, machine learning/data mining models and statistical analysis including linear, multiple, rank, logistics and multinomial regression, ARIMA, density estimation and support vector regression, where Sukhobokov (2018) consider under the category of predictive analytics, implicative analytics and graph analytics models.
Time-series forecasting models can also be grouped under the predictive analysis category of models (Tipi, 2021). There may be particular software packages dealing with this type of analyses, and there is the need to consider dedicated skills to model and use analytics in this case.
Prescriptive analytics
When prescriptive analytics are considered, considerations are not only given to what may happen, but also to what can be done based on what happened. In this case, a solution to a problem is to be identified and proposed ready for implementation.
It is relevant to note that predictive and prescriptive analytics may use similar data as well as they may use similar techniques, however, the purpose of the analytics may be different.
Multi-criteria decision making, optimization, simulations are some of the techniques identified by Wang et al. (2018) where Souza (2014) are including techniques such as Analytic Hierarchy Process (AHP) technique, Game theory (e.g. auction design, contract design), mixed-integer linear programming (MILP), non-linear programming, network flow algorithms, stochastic dynamic programming under this category.
Lepenioti et al. (2020) are also listing a number of prescriptive analytical techniques in their study and some of these are: probabilistic models, machine learning/data mining, mathematical programming models, evolutionary computation models, simulation models and logic-based models (Tipi, 2021, p12).
For example, under mathematical programming, they have captured modelling techniques such as: mixed-integer programming, linear programming, binary quadratic programming, non-linear programming, binary linear integer programming, stochastic optimisation, conditional stochastic optimisation, constrained Bayesian optimisation, fuzzy linear optimisation, robust and adaptive optimisation, dynamic programming and optimal search path.
Many of these models can be directly applied in the field of supply chain. For example: resource allocation, covering, transportation, transshipment models with different distribution stages to name just a few. Under the evolutionary computation, Lepenioti et al. (2020) have listed models such as genetic algorithm, evolutionary optimisation, greedy algorithm, particle swarm optimisation.
In a study of quantitative models for sustainable supply chain, Brandenburg et al. (2014) capture models such as mathematical programming using single and multi-objectives optimisation; simulation methods such as spreadsheets, system dynamics, discrete event and business game; heuristic methods (simple heuristic, artificial intelligence, meta-heuristics); hybrid models; and analytical models using multi-criteria decision making, game theory and systemic models.
In the area of simulation and simulation models used for supply chain modelling, Chilmon and Tipi (2020) have identified the following categories: agent-based simulations, discrete event simulations, system dynamic simulations, Monte Carlo, queuing simulations, analytical models supported by simulations, hybrid simulations, parallel and distributed simulations and object-oriented simulations.
Where the list of models and modelling techniques is extensive, it has been noted that simulations have been used alongside other models in supply chain analysis in the case of modelling an end-to-end supply chain.
It is relevant to note that a hybrid approach, where more than one model or technique is required to capture the complexities of a supply chain, is preferred over a single technique, as there is no single model that can be employed to capture the complexities of a supply chain. Hybrid models among others are also noted in Min and Zhou (2002) and Kabak and Ulengin (2011), where they still appear to be preferred over other dedicated models when working with analysis in the context of supply chain.
As the scope of implementing analytics is not only to seek a reason for what happens but also to indicate a solution, the decision on adopting prescriptive analytics as a tool brings complexity in approach, the data to be considered, the software package to be used, as well as the technique or set of techniques to be implemented.
The argument put forward by Holsapple et al. (2014) for implementing analytics is to give consideration to aspects on obtaining value from data available, creating competitive advantage, generate improvements on an organization’s performance, to have a positive effect on a decision and generate knowledge.
It is relevant to add here that the benefit of adopting analytics should not be seen as a one-off approach, as they should be seen as bring continuous benefits over a period of time.
The aspect of automation in decision-making needs to be taken into consideration. A number of approaches aim to embed considerations of artificial intelligence that allow for incorporation of automation where for example, decisions on operations such as reorder point, or stock availability, are being taken without the need of human interaction. Lepenioti et al. (2020 p. 67) put forward the proposition that “prescriptive analytics can enable decision automation, provided that the challenges of uncertainty, dynamicity and complexity are faced effectively”.
Discussion
A number of challenges are noted when implementing analytics in the supply chain.
Implementing BA within an organization is costly and time-consuming where benefits may not be immediate, and they may only be observed over a longer period of time. There are situations when analytics are used to identify the optimum utilization of resources, or identifying the best routing plan, or the optimum location of a warehouse, where the benefits of implementing these are observed within a shorter period of time.
However, there are situations where an implementable solution may bring short and long-term benefits, but they are not as easily identifiable as they may not be directly captured within a measurement system. This raises the challenge of evaluating the benefits of investing in analytics within an organization and across their supply chain.
Organizations across the supply chain may consider implementing analytics at various levels of complexity, where the real benefit of implementing analytics can only be noted if similar functionality and access to data is available across the chain. For complex, longer supply chains this may not be possible due to data available, or infrastructure, or technology used.
User interaction with analytics is another challenge that needs to be noted here, where particular skills may be required to make use of the technology and software implemented with the adoption of analytics. User interaction is not only limited to the availability of documentation and training, but also to allow for a creative engagement with the task.
However, automation in decision is considered and embedded in many routine operations, the user engagement with technology is expected to be at a “new and different” level of understanding and reflection of what may happen with various activities within an organization and across the supply chain. Therefore, there is an expectation of users and analysts to engage in understanding, using and in some cases developing predictive and prescriptive models for supply chain.
One other challenge that needs to be taken into considerations is the rapid development of technology. Changes in technology may lead to the need to re-evaluate the need for various operations in the supply chain, as well as the structure and flows in the chain.
Conclusion
The use of analytics and in particular prescriptive analytics in the supply chain is not new. However, there are a number of new challenges that can be observed with the increase in the data present in the supply chain as well as new technological developments.
Therefore there is a further need for research in this area. Tipi (2021) has noted areas of research in the supply chain that will directly benefit from the use of prescriptive analytics such as: big data analytics in the supply chain; expert systems; digitalization, digital supply chain; information accuracy, completeness and timeliness; resilience and sustainability in the supply chain; self-thinking supply chain; supply chain 4.0 and others.
Other areas that capture the use of technology are referred the themes such as data science; information and digital technology; challenges in technology adoption; blockchain adoption in the supply chain; enabling technologies; demand forecasting; lifecycle technology; ICT developments with autonomous and predictive capabilities; big data technologies and new technologies.
When implementing BA in organizations and supply chain considerations need to be given to data and big data that is internal and external to an organization and comes in various forms and sizes.
A large library of models employing prescriptive analytics have been developed and successfully implemented in supply chains, still there is no one model or technique that is best used overall to meet the needs of a supply chain. There is a need to further understand how to bring models to work together with the aim to respond to current challenges in the supply chain.
A long list of software packages are available that allow for various aspects to be modelled and implemented, still to be in a position to implement and use these applications, there is the need for users and analysts to gain knowledge not only on software development but also supply chain management to be in a better position to identify creative solutions to supply chain issues.
References
Arunachalam, D, Kumar, N and Kawalek, J P (2018) “Understanding big data analytics capabilities in supply chain management: Unravelling the issues, challenges and implications for practice”, Transportation Research Part E, Vol. 114, pp. 416-436.
Brandenburg, M, Govindan, K, Sarkis, J and Seuring, S (2014) “Quantitative models for sustainable supply chain management: Developments and directions”, European Journal of Operational Research. Vol. 233, pp. 299-312.
Chilmon, B and Tipi, N S (2020) “Modelling and simulation considerations for an end-to-end supply chain system”, Computers & Industrial Engineering, Vol. 150, https://doi.org/10.1016/j.cie.2020.106870
Kabak, O and Ulengin, F (2011) “Possibilistic linear-programming approach for supply chain networking decisions”, European Journal of Operational Research, Vol. 209, pp 253-264.
Holsapple, C, Lee-Post, A and Pakath, R (2014) “A unified foundation for business analytics”, Decision Support Systems, Vol. 64, pp. 130-141. doi:10.1016/j.dss.2014.05.013
Frederico, G F, Garza-Reyes, J A, Anosike, A and Kumar, V (2019) “Supply Chain 4.0: concepts, maturity and research agenda”, Supply chain management, doi:10.1108/SCM-09-2018-0339
Lepenioti, K, Bousdekis, A, Apostolou, D and Mentzas, G (2020) “Prescriptive analytics: Literature review and research challenges”, International Journal of Information Management, Vol. 50, pp. 57-70.
Min, H and Zhou, G (2002) “Supply chain modeling: past, present and future”, Computers & Industrial Engineering, Vol. 43, pp. 231-249.
Sivarajah, U, Kamal, M M, Irani, Z, and Weerakkody, V (2017) “Critical analysis of Big Data challenges and analytical methods”, Journal of Business Research, Vol. 70, pp. 263-286. doi:10.1016/j.jbusres.2016.08.001
Sharda, R, Delen, D, Turban, E, Aronson, J E, Liang, T-P and King, D (2018) Business intelligence, analytics, and data science: a managerial perspective (Fourth, Global ed.). Harlow, England: Pearson.
Sukhobokov, A A (2018) “Business analytics and AGI in corporate management systems”, Procedia Computer Science, Vol. 145, pp. 533-544. doi:10.1016/j.procs.2018.11.118
Souza, G C (2014) “Supply chain analytics”, Business Horizons, Vol. 57, pp. 595-605.
Stanton, W W and Stanton, A D (2020) “Helping Business Students Acquire the Skills Needed for a Career in Analytics: A Comprehensive Industry Assessment of Entry-Level Requirements”, Decision Sciences Journal of Innovative Education, Vol. 18(1), pp. 138-165. doi:10.1111/dsji.12199
Tipi, N. (2021) Supply Chain Analytics and Modelling, Kogan Page 2021, ISBN: 9780749498627, UK.
Wang, C-H, Cheng, H-Y, and Deng, Y-T (2018) “Using Bayesian belief network and time-series model to conduct prescriptive and predictive analytics for computer industries”, Computers & Industrial Engineering, Vol. 115, pp. 486-494. doi:10.1016/j.cie.2017.12.003